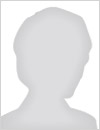
This will count as one of your downloads.
You will have access to both the presentation and article (if available).
In contrast to simple Pearson correlation networks, the partial correlations (PC) only identify direct correlations while indirect associations are eliminated. In addition to this, the state-of-the-art techniques in brain research are based on static graph theory, which is unable to capture the dynamic behavior of the brain connectivity, as it alters with disease evolution. We propose a new research avenue in neuroimaging connectomics based on combining dynamic graph network theory and modeling strategies at different time scales. We present the theoretical framework for area aggregation and time-scale modeling in brain networks as they pertain to disease evolution in dementia. This novel paradigm is extremely powerful, since we can derive both static parameters pertaining to node and area parameters, as well as dynamic parameters, such as system’s eigenvalues. By implementing and analyzing dynamically both disease driven PC-networks and regular concentration networks, we reveal differences in the structure of these network that play an important role in the temporal evolution of this disease. The described research is key to advance biomedical research on novel disease prediction trajectories and dementia therapies.
Analysis of breast MRI data based on (topographic) independent and tree-dependent component analysis
This will count as one of your downloads.
You will have access to both the presentation and article (if available).
View contact details
No SPIE Account? Create one