|
ACCESS THE FULL ARTICLE
No SPIE Account? Create one
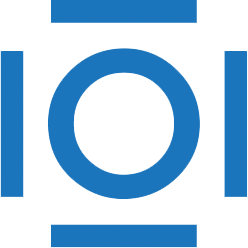
CITATIONS
Cited by 8 scholarly publications.
Neural networks
Earth observing sensors
Satellite imaging
Satellites
Image classification
Vegetation
Data modeling