|
ACCESS THE FULL ARTICLE
No SPIE Account? Create one
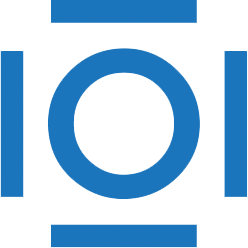
CITATIONS
Cited by 2 scholarly publications.
Error analysis
Analytical research
Feature extraction
Image classification
Network architectures
Computer engineering
Data modeling