|
ACCESS THE FULL ARTICLE
No SPIE Account? Create one
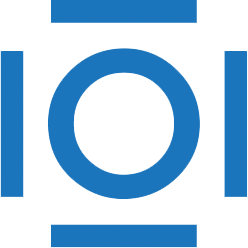
CITATIONS
Cited by 1 patent.
Digital filtering
Maritime surveillance
Video
Video surveillance
Cameras
Image filtering
Detection and tracking algorithms