|
ACCESS THE FULL ARTICLE
No SPIE Account? Create one
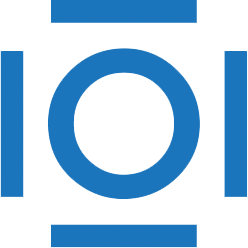
CITATIONS
Cited by 8 scholarly publications.
Image processing
Video
Optical engineering
Fourier transforms
Content addressable memory
Motion models
Principal component analysis