Purpose: We investigated the performance of a neural network (NN) material decomposition method under varying pileup conditions. Approach: Experiments were performed at tube current settings that provided count rates incident on the detector through air equal to 9%, 14%, 27%, 40%, and 54% of the maximum detector count rate. An NN was trained for each count-rate level using transmission measurements through known thicknesses of basis materials (PMMA and aluminum). The NN trained for each count-rate level was applied to x-ray transmission measurements through test materials and to CT data of a rod phantom. Material decomposition error was evaluated as the distance in basis material space between the estimated thicknesses and ground truth. Results: There was no clear trend between count-rate level and material decomposition error for all test materials except neoprene. As an example result, Teflon error was 0.33 cm at the 9% count-rate level and 0.12 cm at the 54% count-rate level for the x-ray transmission experiments. Decomposition error increased with count-rate level for the neoprene test case, with 0.65-cm error at 9% count-rate level and 1.14-cm error at the 54% count-rate level. In the CT study, material decomposition error decreased with increasing incident count rate. For example, the material decomposition error for Teflon was 0.089, 0.066, 0.054 at count-rate levels of 14%, 27%, and 40%, respectively. Conclusions: Results demonstrate over a range of incident count-rate levels that an NN trained at a specific count-rate level can learn the relationship between photon-counting spectral measurements and basis material thicknesses. |
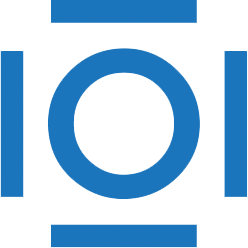
CITATIONS
Cited by 8 scholarly publications.
Sensors
Polymethylmethacrylate
Aluminum
Neural networks
Calibration
X-rays
X-ray computed tomography