Existing methods for detecting surface defects in strip steel are mainly aimed at the case of low-resolution images, and little research has been done in the case of high-resolution images with a small percentage of valid targets. In response to the practical problems in the hot-rolled flattening stage, we propose a hot-rolled strip surface defect dataset called XLData-CLS, which contains a total of 5000 defective samples and 10,000 normal samples with an image resolution of 1344 × 672 pixels. Based on this dataset, this paper improves the ResNet152 model by adding a feature extraction layer at the front of the model so that it can extract information more effectively for high-resolution images. Experimental results on the XLData-CLS dataset show that the proposed method achieves 99.00% classification accuracy on the test set, which is higher than other comparable deep learning models. Validation results on another datasets also demonstrate the accuracy of our method for high-resolution defective images. |
ACCESS THE FULL ARTICLE
No SPIE Account? Create one
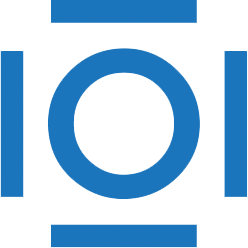
CITATIONS
Cited by 1 scholarly publication.
Defect detection
Data modeling
Feature extraction
Image resolution
Convolution
Statistical modeling
Inspection