The detection of pavement crack information plays an important role in the service evaluation of infrastructure engineering performance. The randomness of road noise, pollution, and spots make it difficult to ensure the accuracy of traditional image processing algorithms to detect cracks. Therefore, the crack identification technology under complex background conditions is particularly important. We propose a network architecture, named feature pyramid and attention enhancement network (FPAEN) for pavement crack detection. Through a new multiscale feature fusion module, the deep semantic information is integrated into the low-level convolution stage layer by layer, so the context information circulates throughout the whole network. In addition, the attention enhancement mechanism and the multiscale dilated convolution module are used to strengthen the network model’s ability to locate the crack pixels. To evaluate the performance of the proposed approach, we train FPAEN on the DeepCrack dataset and test it on four datasets. Experimental results show that our method outperforms other advanced crack detection approaches in F-score and mean intersection over union. |
ACCESS THE FULL ARTICLE
No SPIE Account? Create one
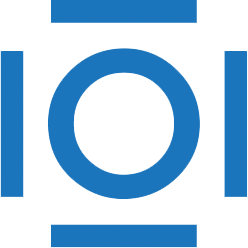
CITATIONS
Cited by 3 scholarly publications.
Convolution
Image enhancement
Network architectures
Convolutional neural networks
Detection and tracking algorithms
Image processing
Roads