Semantic segmentation of three-dimensional (3D) scenes is a challenging task in 3D scene understanding. Recently, deep learning-based segmentation approaches have made significant progress. A multiscale spatial context feature learning is used in an end-to-end approach for 3D point cloud semantic segmentation. Furthermore, a local feature fusion learning block is then introduced to the hidden layers in the network to improve its feature learning capability. In addition, features learned in several different layers are fused for further improvement. Based on these strategies, end-to-end architectures are finally designed for 3D point cloud semantic segmentation. Several experiments conducted on three publicly available datasets have clearly shown the effectiveness of the proposed network. |
ACCESS THE FULL ARTICLE
No SPIE Account? Create one
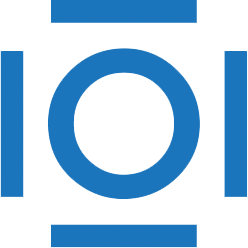
CITATIONS
Cited by 1 scholarly publication.
Clouds
Image segmentation
3D image processing
Feature extraction
RGB color model
3D modeling
Neural networks