|
ACCESS THE FULL ARTICLE
No SPIE Account? Create one
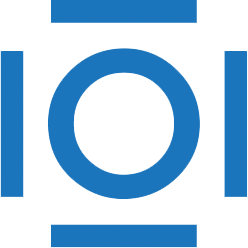
CITATIONS
Cited by 1 scholarly publication.
Sensors
Neural networks
Quantization
Lithium
Image processing
Head
Network architectures