|
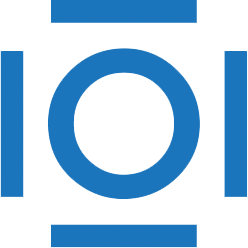
CITATIONS
Cited by 3 scholarly publications.
Chemical species
Associative arrays
Databases
Facial recognition systems
Detection and tracking algorithms
Image classification
Target recognition