Remote sensing scene classification has received more and more attention as important fundamental research in recent years. However, the redundant background information and complex spatial scale variability of remote sensing scene images make the existing convolutional neural network models, which mainly concentrate on global features, perform poorly. To effectively alleviate these problems, we proposed an MSRes-SplitNet model based on multiscale features and attention mechanisms for remote sensing scene image classification. First, MSRes blocks are constructed for the extraction of multi-scale features. Then, the multi-channel local features are fused by the Split-Attention block. Finally, the global and local feature information is aggregated by convolution, thus obtaining multi-scale features while alleviating the small-sample learning problem. Experiments are conducted on three publicly available datasets and compared with other state-of-the-art methods, showing that the proposed method MSRes-SplitNet has better performance while effectively reducing a large number of parameters. |
ACCESS THE FULL ARTICLE
No SPIE Account? Create one
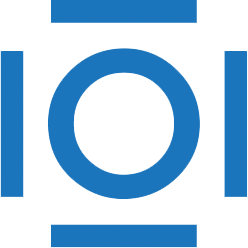
CITATIONS
Cited by 1 scholarly publication.
Remote sensing
Image classification
Convolution
Feature extraction
Performance modeling
Scene classification
Curium