Mercury (Hg) is a heavy metal pollutant characterized by global migration, high bioaccumulation, and high biological toxicity. Soil Hg pollution seriously harms soil environmental quality and human health. The higher the content of Hg in the soil, the more serious the harm. Therefore, the acquisition of soil Hg content information is crucial for soil monitoring and restoration, and human health. The purpose of this study is to establish the best hyperspectral prediction model of soil Hg content in the study area. A total of 90 soil samples were collected from the mining areas and suburbs, and the Hg content was analyzed. Several preprocessing methods were applied to the hyperspectral data. Partial least-squares regression (PLSR), orthogonalized PLSR, random forest, and back propagation neural network (BPNN) were used to establish the relationship between soil hyperspectral data and soil Hg content to predict the soil Hg content. Specifically, the transferability of the best prediction model in yellow-brown and cinnamon soil data was discussed. The results showed that BPNN + standard normal variable + full-band (4 nm) is the optimum method to predict soil Hg content ( |
ACCESS THE FULL ARTICLE
No SPIE Account? Create one
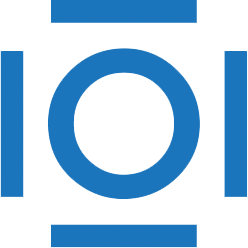
CITATIONS
Cited by 1 scholarly publication.
Mercury
Data modeling
Soil science
Soil contamination
Metals
Machine learning
Minerals