Vehicle detection is an important method for understanding high-resolution remote sensing images. Deep convolutional neural network (DCNN)-based methods have improved many computer vision tasks and have achieved state-of-the-art results in many object detection datasets. Object detection of remote sensing images has been radically changed by the introduction of DCNN. Considering correlation between the scale distribution of objects and spatial resolution of remote sensing images, we propose an improved vehicle detection method based on a YOLOv3 model. A multi-scale clustering anchor box generation algorithm is proposed to obtain the anchor box parameters that match the resolution of each layer of the feature pyramid of model. This allows us to get more accurate anchor parameters. Focal loss is introduced into the default loss function to reduce the weight of negative samples, which were easily classified, that focus the model training process on samples that are difficult to classify. For the imbalance problem of positive and negative samples in the detection method based on the prior anchor box, focal loss is used to focus the model training process on samples that are difficult to classify. The experiment is performed on a dataset consisting of remote sensing images obtained from Worldview-3, and the results show that compared with the basic YOLOv3 algorithm, the average accuracy of vehicle detection is improved by 8.44%. The accuracy of vehicle detection of high-resolution remote sensing images is significantly improved while maintaining the speed of single-stage target detection. This approach is tested on an xView dataset consisting of remote sensing images obtained from Worldview-3. In addition, through using the proposed method, the average precision of vehicle detection increased by 8.44%. The experimental results show that the proposed method can be used for object detection in high-resolution remote sensing images effectively, and this method can significantly improve the performance of the model without sacrificing inference speed. |
ACCESS THE FULL ARTICLE
No SPIE Account? Create one
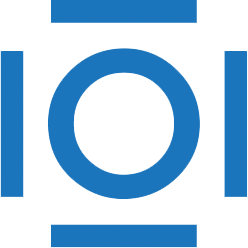
CITATIONS
Cited by 11 scholarly publications.
Remote sensing
Performance modeling
Target detection
Statistical modeling
Data modeling
Process modeling
Head