The use of ground truth (GT) data in the learning and/or assessment of classification algorithms is essential. Using a biased or simplified GT attached to a remote sensing image to partition does not allow a rigorous explanation of the physical phenomena reflected by such images. Unfortunately, this scientific problem is not always treated carefully and is generally neglected in the relevant literature. Furthermore, the impacts of obtained classification results for decision-making are negative. This is inconsistent when considering investments in both the development of sophisticated sensors and the design of objective classification algorithms. Any GT must be validated according to a rigorous protocol before utilization, which is unfortunately not always the case. The evidence of this problem is provided, using two popular hyperspectral images (Indian Pine and Pavia University) that misleadingly are frequently used without care by the remote sensing community since the associated GTs are not accurate. The heterogeneity of the spectral signatures of some GT classes was proven using a semisupervised and an unsupervised classification method. Through this critical analysis, we propose a general framework for a minimum objective assessment and validation of the GT accuracy, before exploiting them in a classification method. |
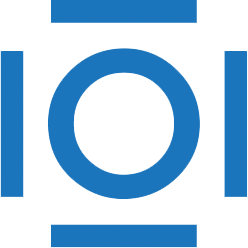
CITATIONS
Cited by 7 scholarly publications.
Image visualization
Hyperspectral imaging
Visualization
Remote sensing
Image classification
Algorithm development
Sensors