|
ACCESS THE FULL ARTICLE
No SPIE Account? Create one
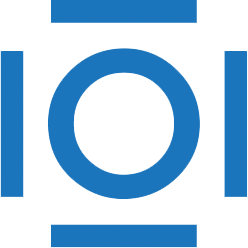
CITATIONS
Cited by 4 scholarly publications.
Floods
Error analysis
Lithium
Satellites
Algorithm development
Analytical research
Image classification