|
ACCESS THE FULL ARTICLE
No SPIE Account? Create one
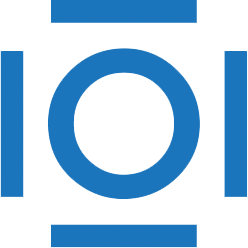
CITATIONS
Cited by 4 scholarly publications.
Video
Video
Data modeling
Video surveillance
Video surveillance
Feature extraction
Video processing