Imperviousness index map generation was a two-stage procedure. The first step was classification, which divided the study area into two categories: a) completely permeable (imperviousness index less than 1%) and b) fully or partially impervious areas. For pixels classified as impervious, the percentage of impervious surface coverage within a single pixel was estimated. Decision and regression trees models construction was done based on training data set derived from Landsat TM pixels as well as for fragments of RapidEye images corresponding to the same Landsat TM training pixels. In order to obtain imperviousness index maps with the minimum possible error we did the estimation of models accuracy based on the results of cross-validation. The approaches guaranteeing the lowest means errors in terms of training set using C5.0 and Cubist algorithm for Landsat and RapidEye images were selected. Accuracy of the final imperviousness index maps was checked based on validation data sets. The root mean square error of determination of the percentage of the impervious surfaces within a single Landsat pixel was 9.9% for C.5.0/Cubist method. However, the root mean square error specified for RapidEye test data was 7.2%. The study has shown that better results of two-stage imperiousness index map estimation using RapidEye satellite images can be obtained. |
ACCESS THE FULL ARTICLE
No SPIE Account? Create one
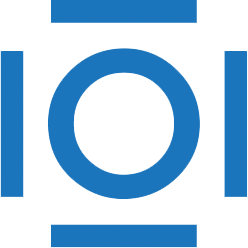
CITATIONS
Cited by 1 scholarly publication.
Earth observing sensors
Landsat
Image resolution
Error analysis
Image classification
Satellites
Satellite imaging