|
ACCESS THE FULL ARTICLE
No SPIE Account? Create one
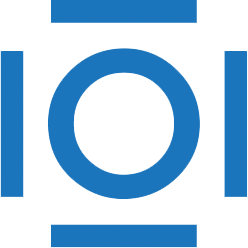
CITATIONS
Cited by 3 scholarly publications.
Earth observing sensors
Landsat
Data modeling
Atmospheric modeling
Remote sensing
Hyperspectral imaging
Algorithm development