|
ACCESS THE FULL ARTICLE
No SPIE Account? Create one
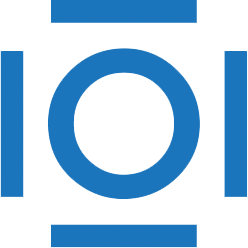
CITATIONS
Cited by 3 scholarly publications.
Wavelets
Signal processing
Denoising
Image processing
Image segmentation
Matrices
Social networks