|
ACCESS THE FULL ARTICLE
No SPIE Account? Create one
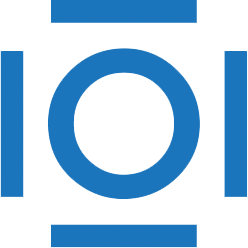
CITATIONS
Cited by 1 scholarly publication.
Magnetorheological finishing
Digital filtering
Machine learning
RGB color model
Active remote sensing
Image fusion
Optical filters