|
ACCESS THE FULL ARTICLE
No SPIE Account? Create one
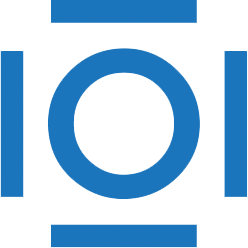
CITATIONS
Cited by 4 scholarly publications.
Breast
Tissues
Mammography
Feature extraction
Image segmentation
Breast cancer
Databases