|
ACCESS THE FULL ARTICLE
No SPIE Account? Create one
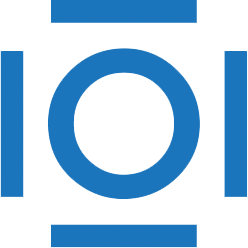
CITATIONS
Cited by 4 scholarly publications.
Error analysis
Sensors
Matrices
Statistical analysis
Chemical elements
Filtering (signal processing)
Modeling