|
ACCESS THE FULL ARTICLE
No SPIE Account? Create one
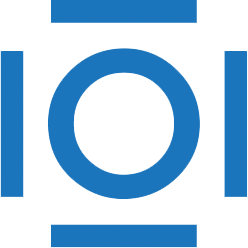
CITATIONS
Cited by 10 scholarly publications.
Principal component analysis
Speaker recognition
Expectation maximization algorithms
Databases
Performance modeling
Data modeling
Statistical analysis