|
ACCESS THE FULL ARTICLE
No SPIE Account? Create one
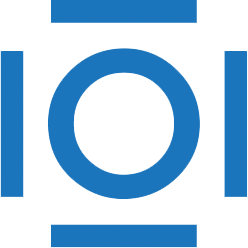
CITATIONS
Cited by 1 scholarly publication.
General packet radio service
Land mines
Detection and tracking algorithms
Data fusion
Feature extraction
Metals
Performance modeling