|
ACCESS THE FULL ARTICLE
No SPIE Account? Create one
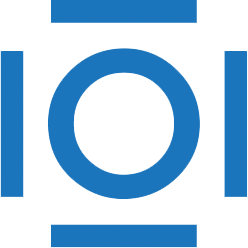
CITATIONS
Cited by 1 scholarly publication.
Gaussian filters
Filtering (signal processing)
Surveillance
Nonlinear filtering
Electronic filtering
Data modeling
Optimization (mathematics)