|
ACCESS THE FULL ARTICLE
No SPIE Account? Create one
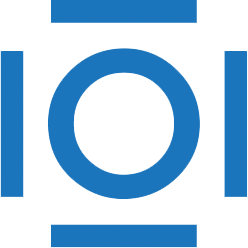
CITATIONS
Cited by 4 scholarly publications.
Principal component analysis
Databases
Facial recognition systems
Classification systems
Data modeling
Systems modeling
Technetium