|
ACCESS THE FULL ARTICLE
No SPIE Account? Create one
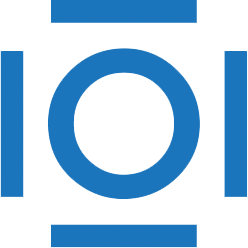
CITATIONS
Cited by 3 scholarly publications.
Image segmentation
Image processing algorithms and systems
Magnetorheological finishing
Cervix
Tissues
Expectation maximization algorithms
Feature extraction