|
ACCESS THE FULL ARTICLE
No SPIE Account? Create one
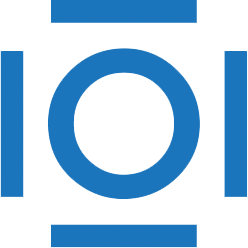
CITATIONS
Cited by 1 scholarly publication.
Stars
Feature selection
Astronomy
Observatories
Databases
Astrophysics
Galactic astronomy