|
ACCESS THE FULL ARTICLE
No SPIE Account? Create one
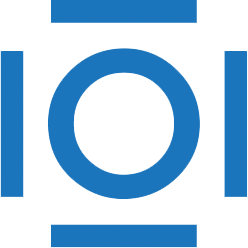
CITATIONS
Cited by 4 scholarly publications.
Principal component analysis
Independent component analysis
Feature extraction
Automatic target recognition
Image filtering
Target recognition
Target detection