|
ACCESS THE FULL ARTICLE
No SPIE Account? Create one
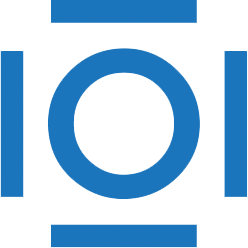
CITATIONS
Cited by 5 scholarly publications.
Databases
Dysprosium
Matrices
Principal component analysis
Data analysis
Data centers
Data processing