|
ACCESS THE FULL ARTICLE
No SPIE Account? Create one
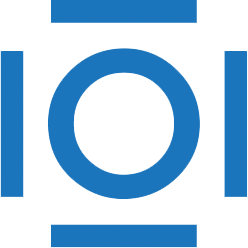
CITATIONS
Cited by 1 scholarly publication.
Data modeling
Ruthenium
Performance modeling
Modeling
Control systems
Bayesian inference
Detection and tracking algorithms