|
ACCESS THE FULL ARTICLE
No SPIE Account? Create one
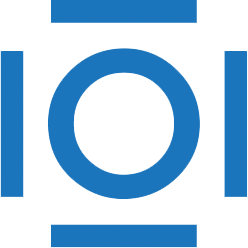
CITATIONS
Cited by 2 scholarly publications.
Principal component analysis
Neural networks
Image classification
Multispectral imaging
Remote sensing
Vegetation
Feature extraction