|
ACCESS THE FULL ARTICLE
No SPIE Account? Create one
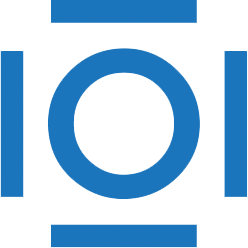
CITATIONS
Cited by 5 scholarly publications.
Photonic integrated circuits
Principal component analysis
Independent component analysis
Algorithm development
Target detection
Data processing
Data compression