|
ACCESS THE FULL ARTICLE
No SPIE Account? Create one
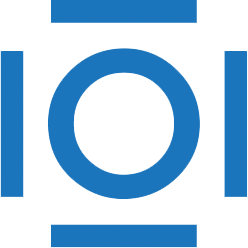
CITATIONS
Cited by 5 scholarly publications.
Hyperspectral imaging
Land mines
Environmental sensing
Explosives
Probability theory
Sensor calibration
Target detection