|
ACCESS THE FULL ARTICLE
No SPIE Account? Create one
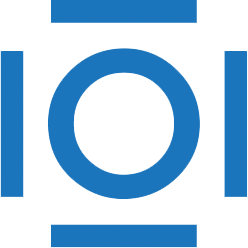
CITATIONS
Cited by 18 scholarly publications.
Lung
Tissues
Wavelets
Image classification
Feature extraction
Machine learning
Computer aided diagnosis and therapy