|
ACCESS THE FULL ARTICLE
No SPIE Account? Create one
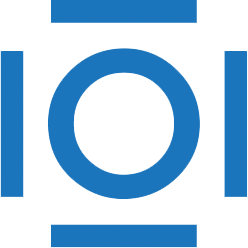
CITATIONS
Cited by 1 scholarly publication.
Statistical modeling
Statistical analysis
Error analysis
Data modeling
Global Positioning System
Estimation theory
Atmospheric modeling