|
ACCESS THE FULL ARTICLE
No SPIE Account? Create one
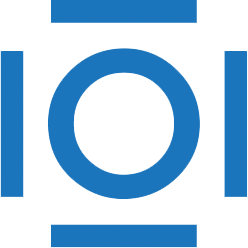
CITATIONS
Cited by 2 scholarly publications.
Data modeling
Electronic signals intelligence
Data fusion
Sensors
Decision support systems
Probability theory
Systems modeling