|
ACCESS THE FULL ARTICLE
No SPIE Account? Create one
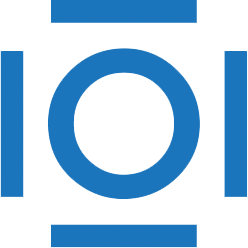
CITATIONS
Cited by 39 scholarly publications and 1 patent.
Image compression
Hyperspectral imaging
Factor analysis
Image quality standards
Remote sensing
Composites
Data compression