|
ACCESS THE FULL ARTICLE
No SPIE Account? Create one
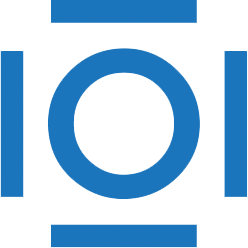
CITATIONS
Cited by 2 scholarly publications.
Imaging systems
Data modeling
Image compression
Sensors
Statistical analysis
Algorithm development
Expectation maximization algorithms