|
ACCESS THE FULL ARTICLE
No SPIE Account? Create one
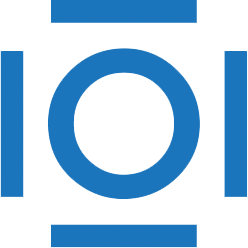
CITATIONS
Cited by 6 scholarly publications.
Sensors
Data modeling
Detection and tracking algorithms
Vegetation
Visualization
Statistical modeling
Algorithm development