|
ACCESS THE FULL ARTICLE
No SPIE Account? Create one
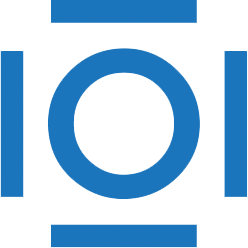
CITATIONS
Cited by 2 scholarly publications and 1 patent.
Simulation of CCA and DLA aggregates
Canonical correlation analysis
Hyperspectral imaging
Sensors
Detection and tracking algorithms
Target detection
Nonlinear dynamics