|
ACCESS THE FULL ARTICLE
No SPIE Account? Create one
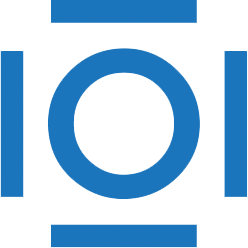
CITATIONS
Cited by 1 patent.
Principal component analysis
Image segmentation
Shape analysis
Binary data
Computer vision technology
Image processing
Image processing algorithms and systems