|
ACCESS THE FULL ARTICLE
No SPIE Account? Create one
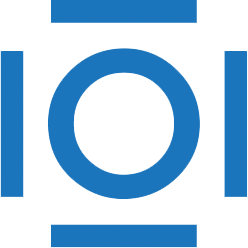
CITATIONS
Cited by 4 scholarly publications.
Interference (communication)
Deconvolution
Inverse problems
Systems modeling
Mathematics
Statistical modeling
System integration