|
ACCESS THE FULL ARTICLE
No SPIE Account? Create one
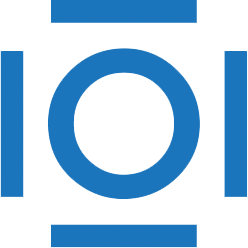
CITATIONS
Cited by 16 scholarly publications.
Image compression
Independent component analysis
Hyperspectral imaging
Principal component analysis
Signal to noise ratio
Image classification
3D image processing