|
ACCESS THE FULL ARTICLE
No SPIE Account? Create one
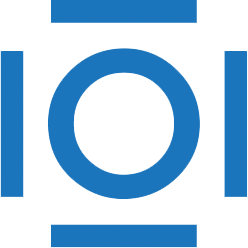
CITATIONS
Cited by 19 scholarly publications.
Sensors
Sensor networks
Data modeling
Machine learning
Detection and tracking algorithms
Acoustics
Environmental monitoring