|
ACCESS THE FULL ARTICLE
No SPIE Account? Create one
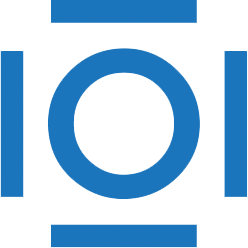
CITATIONS
Cited by 30 scholarly publications and 4 patents.
Image segmentation
3D image processing
Image processing algorithms and systems
Computed tomography
Detection and tracking algorithms
Medical imaging
In vivo imaging