|
ACCESS THE FULL ARTICLE
No SPIE Account? Create one
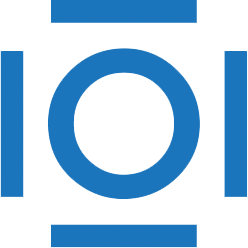
CITATIONS
Cited by 1 scholarly publication.
Video
Semantic video
Feature extraction
Optical tracking
Signal detection
Digital filtering
Machine learning