|
ACCESS THE FULL ARTICLE
No SPIE Account? Create one
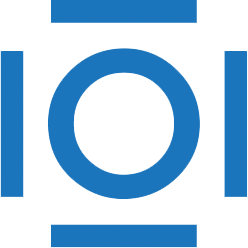
CITATIONS
Cited by 17 scholarly publications.
Filtering (signal processing)
Electronic filtering
Velocity measurements
Nonlinear filtering
Error analysis
Matrices
Performance modeling