|
ACCESS THE FULL ARTICLE
No SPIE Account? Create one
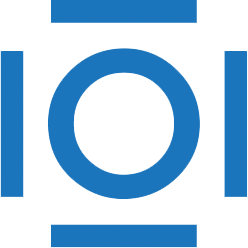
CITATIONS
Cited by 1 scholarly publication.
Image classification
Buildings
Earth observing sensors
Roads
Applied physics
High resolution satellite images
Reflectivity